Oxford researchers develop new AI to enable autonomous vehicles to adapt to challenging weather conditions
Thursday 8th Sep 2022, 12.37pm
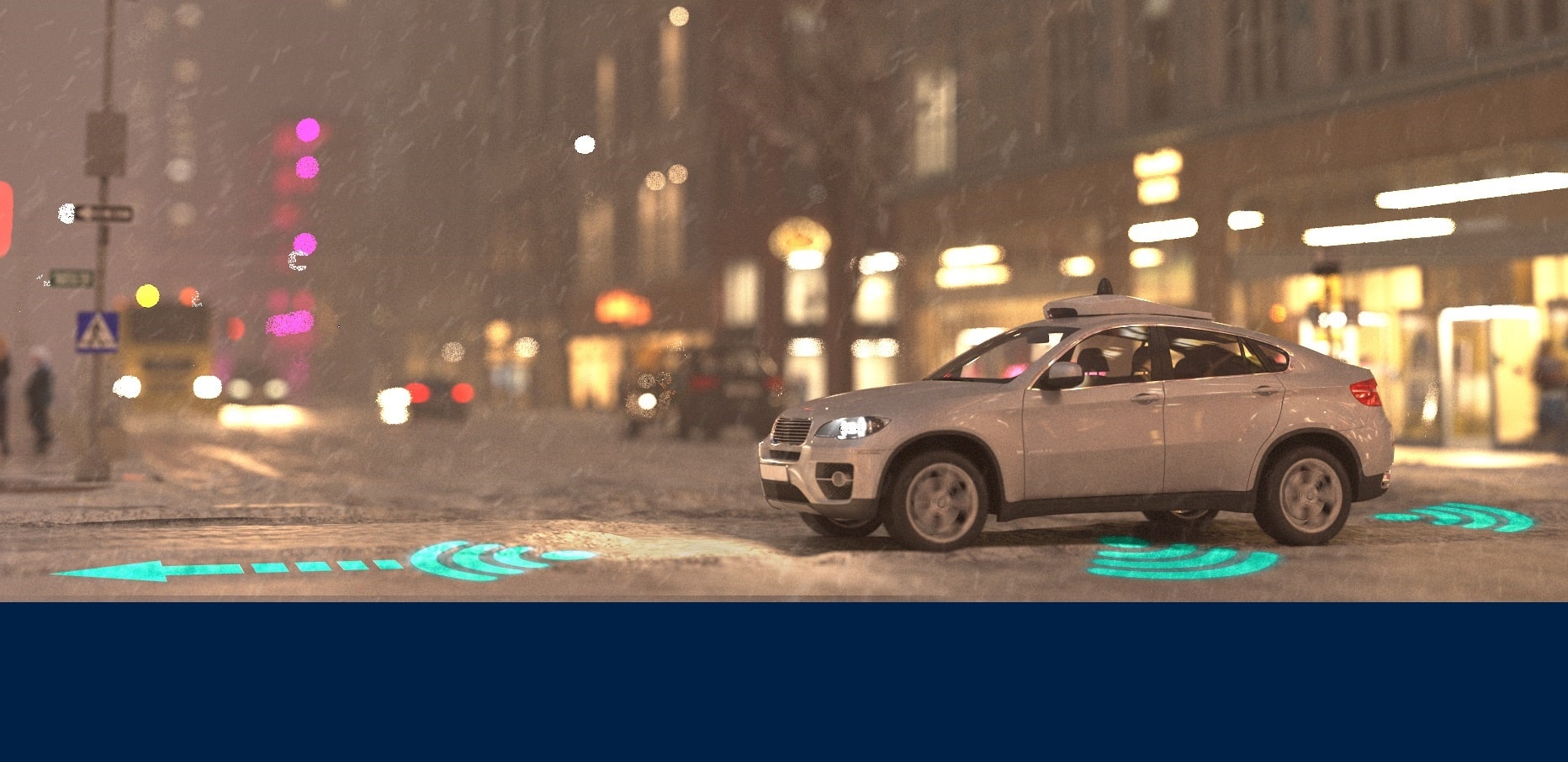
Yasin Almalioglu, who completed the research as part of his DPhil in the Department of Computer Science, said: ‘The difficulty for AVs to achieve precise positioning during challenging adverse weather is a major reason why these have been limited to relatively small-scale trials up to now. For instance, weather such as rain, fog, or snow may cause an AV to detect itself in the wrong lane before a turn, or to stop too late at an intersection because of imprecise positioning.’
To overcome this problem, Almalioglu and his colleagues developed a novel, self-supervised deep learning model for ego-motion estimation, a crucial component of an AV’s driving system that estimates the car’s moving position relative to objects observed from the car itself. The model brought together richly-detailed information from visual sensors (which can be disrupted by adverse conditions) with data from weather-immune sources (such as radar), so that the benefits of each can be used under different weather conditions.
The model was trained using several publicly available AV datasets which included data from multiple sensors such as cameras, lidar, and radar under diverse settings, including variable light/darkness and precipitation. These were used to generate algorithms to reconstruct scene geometry and calculate the car’s position from novel data. Under various test situations, the researchers demonstrated that the model showed robust all-weather performance, including conditions of rain, fog, and snow, as well as day and night.
Estimating the precise location of AVs is a critical milestone to achieving reliable autonomous driving under challenging conditions. This study effectively exploits the complementary aspects of different sensors to help AVs navigate in difficult daily scenarios
Professor Andrew Markham, Department of Computer Science, Oxford University
The team anticipate that this work will bring AVs one step closer to safe and smooth all-weather autonomous driving, and ultimately a broader use within societies.
Professor Niki Trigoni, from the Department of Computer Science at Oxford, who co-supervised the study with Professor Andrew Markham, said: ‘The precise positioning capability provides a basis for numerous core functionalities of AVs such as motion planning, prediction, situational awareness, and collision avoidance. This study provides an exciting complementary solution for the AV software stack to achieve this capability.’
The full paper, Deep learning-based robust positioning for all-weather autonomous driving, is published in Nature Machine Intelligence.